Introduction
Cancer remains one of the most challenging and prevalent diseases affecting millions of lives worldwide. According to IARC WHO around 20 million new cancer cases and 10 million cancer deaths were registered in 2022. Lung, breast, colorectal, prostate, and stomach cancers are the most common types, together accounting for almost half of all the new cases. [1]
.png)
The new cases increase can be attributed to the overall aging of the population [2] as cancer is more common but not exclusive to older adults [3]. It can be diagnosed across all age groups.
Survival rates for cancer patients significantly depend on the timing of cancer detection – the earlier, the better. However, approximately half of all cancers are still only identified in the advanced stages [4].
The need for innovative and swift solutions to diagnose and provide correct treatment early, before cancer spreads or even forms, has led to the integration of ArtificialIntelligence (AI) in the field of oncology. With its ability to process vast amounts of data and identify complex patterns, AI has become a revolutionary tool in cancer research, diagnostics, and therapy.
Applications
1.Diagnostics
One of the most promising applications of AI in the field of oncology is image analysis for early cancer detection. Various diagnostic methods, including computed tomography (CT) scans, magnetic resonance imaging (MRI), mammograms, are used to identify signs of cancer.
AI capabilities can be leveraged to analyze medical images to identify subtle abnormalities that might go unnoticed by human eyes therefore improving cancer screening and enabling earlier diagnosis and intervention.
For example, Kheiron Medical Technologies and Imperial College London has developed a new AI tool called Mia, which can identify up to 13% more breast cancers than humans during screenings. In a study of 25,065 women's mammograms, Mia spotted subtler signs of cancerous tissue that humans had missed. About 83% of these additional cancers were invasive, highlighting Mia’s ability to detect cancers crucial for early intervention. [5]
AI can assist radiologists not only in detecting and classifying cancer, but also making precise delineation of tumor boundaries, assessing treatment response and identifying disease relapse.
2. Cancer research
Scientists employ AI to deepen their understanding of oncogenesis and its progression to further apply these findings in tailoring existing cancer treatments to individual patients and discover new drugs.
Various factors, like DNA repair defects or environmental exposures, may contribute to the accumulation of mutations in the genome, leading to tumor development. These mutations can create distinct patterns known as mutational signatures.
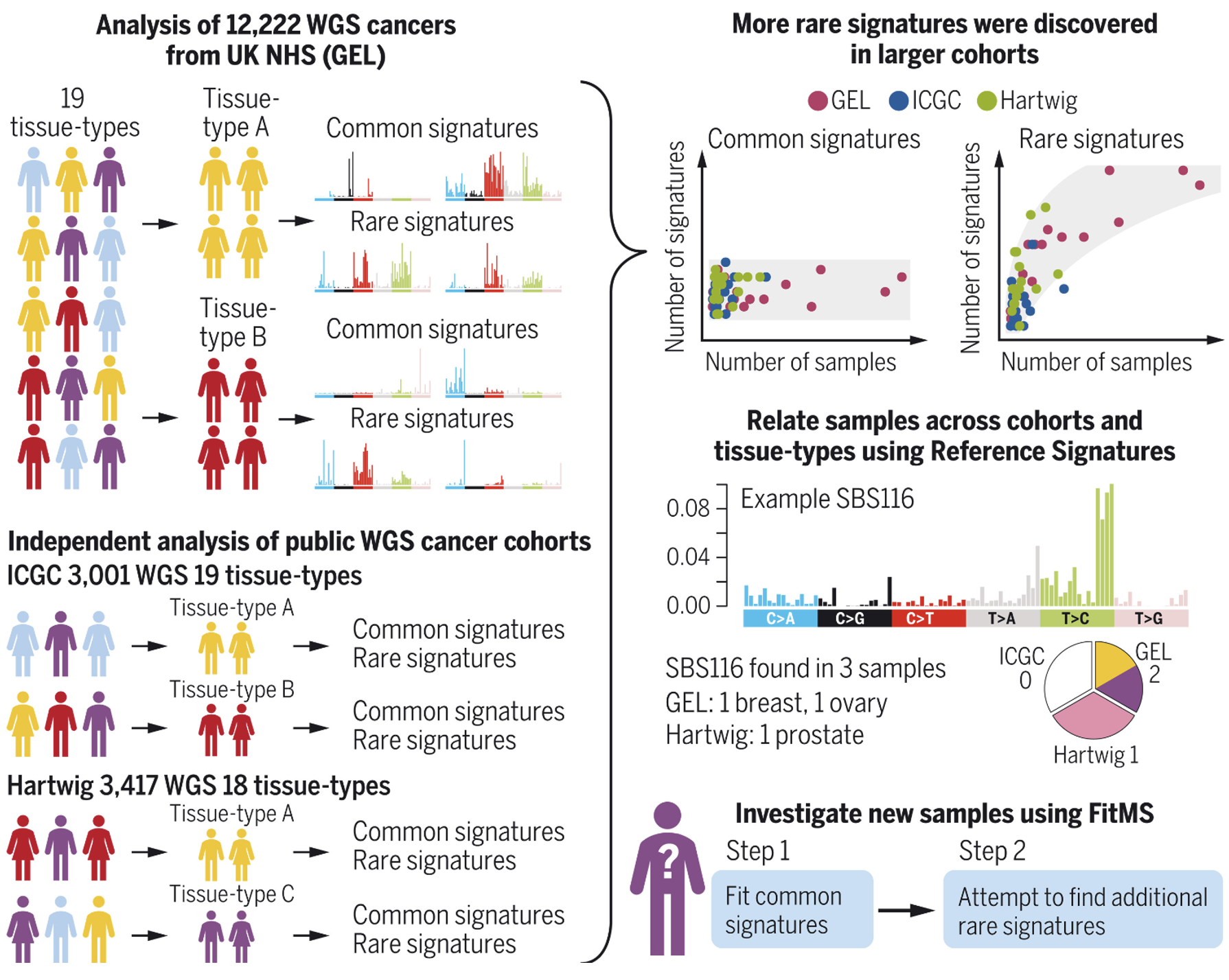
In a recent analysis of 18,640 whole-genome-sequenced cancer samples, researchers at the University of Cambridge identified 58 previously unreported signatures, validated 51 mutational signatures reported earlier, and introduced the concept of common and rare signatures specific to each tumor type. The FitMulti-Step algorithm has been designed to accurately estimate signature exposures. Initially, it identifies common organ-specific signatures and then uncovers additional rare signatures in new samples [6].
These findings enable scientists to identify cancer-associated targets, which is essential in improvement personalized treatment strategies and new drug discovery.
The traditional drug discovery process is time-consuming and costly. AI helps to accelerate this process by analyzing massive datasets to identify potential drug candidates more efficiently. Machine learning algorithms can predict the effectiveness of new compounds, significantly reducing the time and resources required for drug development.
3. Personalized medicine
By analyzing a patient’s genetic and molecular data, AI can identify specific mutations or biomarkers associated with cancer. This information helps oncologists design personalized treatment plans, optimizing the effectiveness of therapies while minimizing side effects.
For patients with advanced cancers, doctors often employ a combination of treatments, including surgery, radiation, and medication. Combining different drugs can enhance treatment effectiveness and reduce side effects. Yet, experimenting with drug combinations is a slow and expensive process.Researchers from Aalto University, University of Helsinki, and University ofTurku have developed a machine learning method to identify the optimal drug combinations, selectively targeting cancer cells based on specific genetic or functional traits. [7].
In immunotherapy, AI can be used to find patterns in the immune system linked to responses and predict how patients will respond to treatment. This helps with patient selection, optimizing treatment, and predicting personalized prognosis.
4. Predictive analytics
Cancer progression can be unpredictable, but AI is significantly improving our ability to forecast the course of the disease in terms of three main parameters - susceptibility, recurrence and mortality.
Machine learning models can analyze patient data, including genetic information, treatment history, and lifestyle factors, to forecast and help healthcare professionals to anticipate potential complications, optimize treatment strategies, and improve overall patient outcomes.
For example, a team of IBM researchers employed a novel deep learning algorithm analyzing mammogram data and patients’ electronic health records to predict malignant breast cancer development within a year. The model achieved an 87% accuracy in forecasting breast cancer development [8].
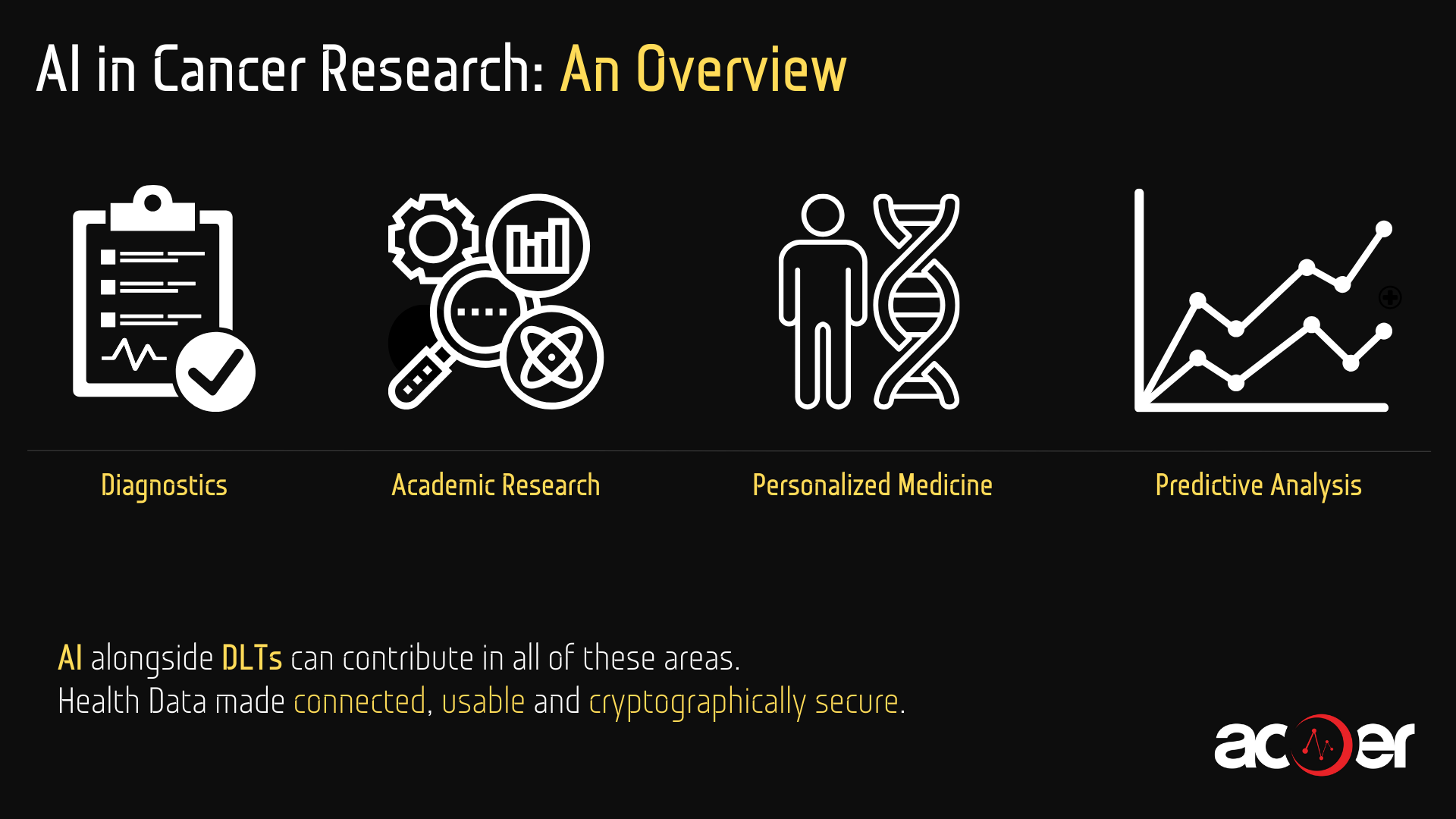
AI and DLT
In all of the use-cases discussed above, the efficacy and success of AI models in cancer research are deeply tied to the quality and integrity of the data they are trained on. This is an area where Distributed Ledger Technologies (DLTs) can help a great deal. DLTs could be used to ensure the verifiability and authenticity of data across multiple stages of AI model development, from initial research to clinical applications. By providing a secure and immutable record of data, DLTs enhance trust in AI's predictive power and the reliability of personalized treatment plans, ultimately supporting the goal of improved patient outcomes in the fight against cancer. Coupling two of the most groundbreaking technologies of the 20th century, AI and DLTs, truly has the potential to transform cancer research as we know it today.